Watch this week's video on YouTube
This week, I want to share my process for analyzing Twitter. Specifically, I want to find who all of my friends follow on Twitter that I don't currently follow. Essentially, I want to build a Twitter follower recommendation engine.
Let's start with the theory behind the problem I'm trying to solve. On Twitter, I follow a bunch of people. Around 450 at the time of filming this video.
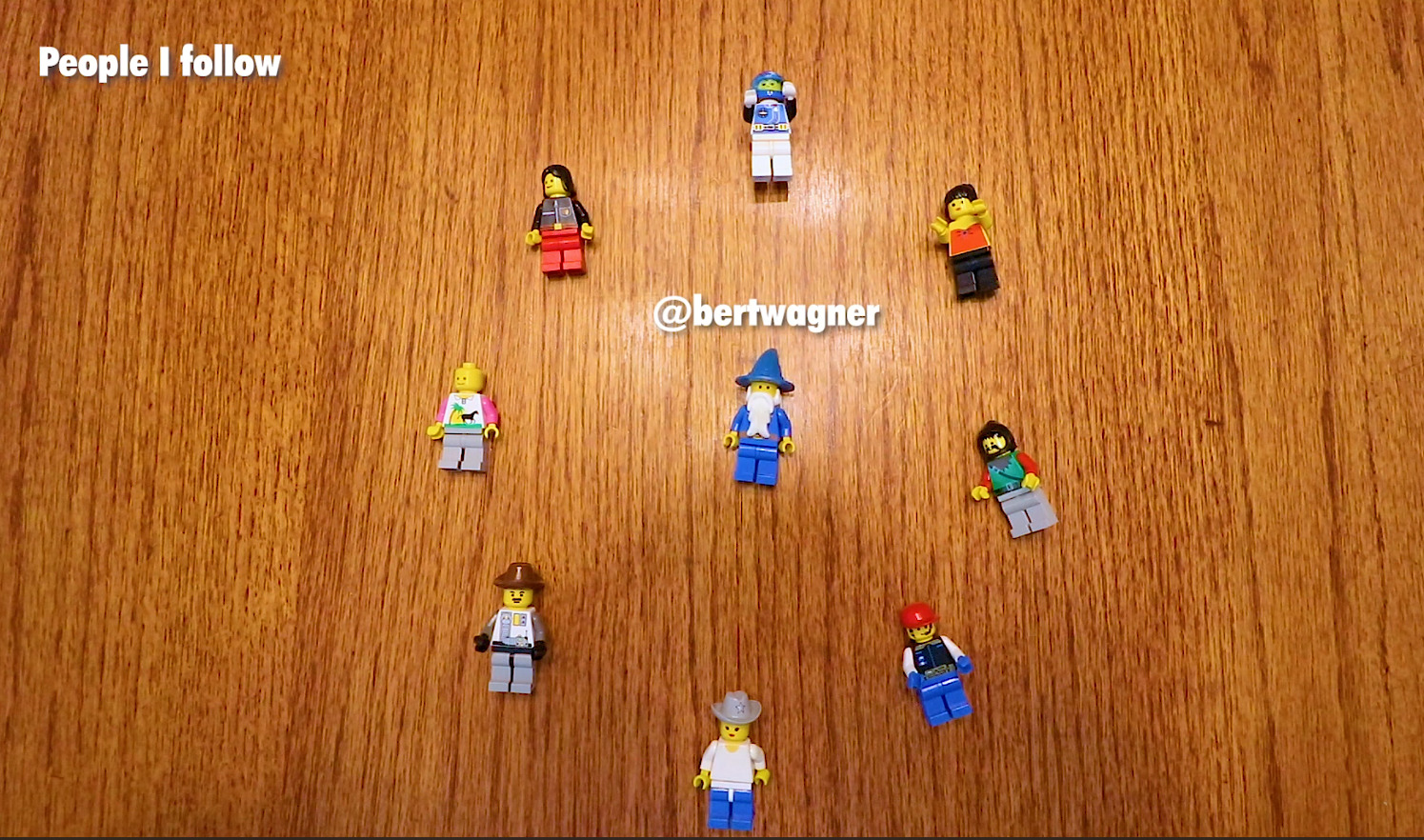
These are people I've either met in person and want to keep in touch with or am interested in following - or both!
Now I don't attend many conferences and events, so finding more people to follow that way is slow going; I probably only meet a handful of new people every year.
However, the second category of people, the interesting ones out there on the internet, is almost limitless. The problem is finding them in a virtual sea of bots, marketing-only accounts, and complainers.
Twitter does have a "Who to Follow" page, and while I'm sure it has some great suggestions, I don't necessarily trust all of Twitter's recommendations. It's kind of like trusting a site like Yelp for restaurant reviews when the local McDonalds rates better than your favorite burger place. I'm sure that particular McDonalds is beautiful by fast food standards, but I'm looking for more personalized recommendations.
So that leads me to this project: I decided to find better personalized recommendations on Twitter by looking at who my friends are following that I am not.
Getting the Data
To start, I needed a list of the people I follow on Twitter, and then a list of who they follow. The proper way to do this is to use the official Twitter API, so I wrote some Python code to do exactly that. Twitter calls the people you follow "Friends", which is funny since I usually don't consider one-way relationships friendships.

I won't go through the code in detail (you can download it from GitHub if you'd like to do that) but essentially it uses the friends/list endpoint to download all of my Friends. I then went through each of those Friends and found all of their Friends.
In total, this ended up being around 125,000 people.
As a quick side note, the Twitter API is terrible. Using it is fine, but the rate limiting is horrendous. I was basically capped at downloading 12,000 people per hour, which meant it took about a day to download all of the data I needed.
Graph Analysis with NetworkX
Once I had the data downloaded, it was time to find relationships between my friends and the people they follow. For this, I decided to use an open source Python library called NetworkX. NetworkX helps perform complex network analysis, which is perfect for what I was trying to do.
NetworkX uses a graph structure to help with its analysis. A graph is made up of of nodes and edges. In our case, the Twitter users are our nodes, and our edges are the relationships. The first thing I did was load all of the people I follow and created a directional edge (aka. an arrow) to indicate the one-way relationship from me to them. Next, I looped over all of those people's friends, adding additional nodes and edges.
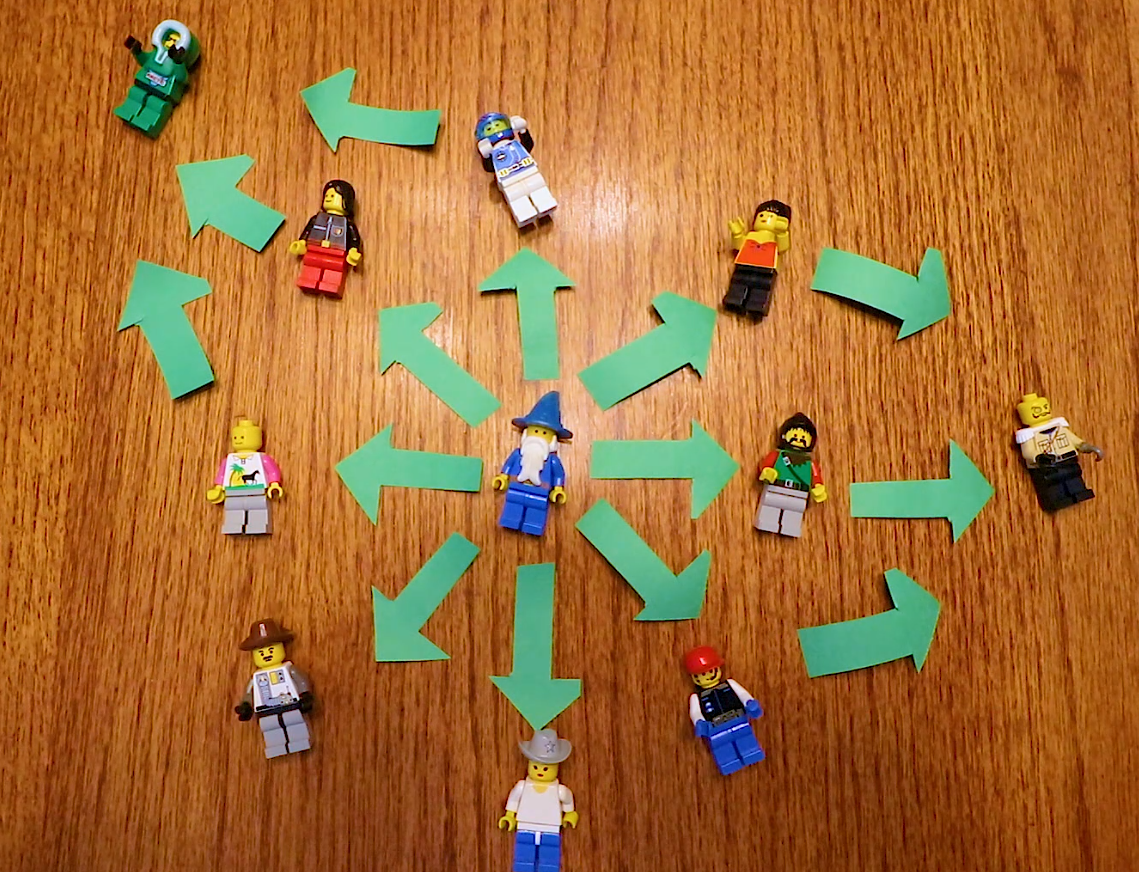
At this point, my graph had 125,000 nodes which was way too many to draw quickly on my computer, and way too many to model with Lego minifigs. I figured not ALL of this data would be useful and I needed a way to filter it.
I started by filtering out any of my friends' friends who happen to already be my friends - this wouldn't be useful since I already follow these people.
Next, I decided to keep only the top 50 most followed people in that second tier of Twitter users. This would limit the processing needed while still giving me a list of the most followed Twitter users that my friends follow that I don't currently follow. Once again, all of this code is available on my GitHub.
At this point, I was able to use NetworkX's drawing capabilities to beautifully render the network of users and relationships. Sure, I could have just listed out the top users that I don't follow, but there is something special about being able to create a visual network of those relationships.
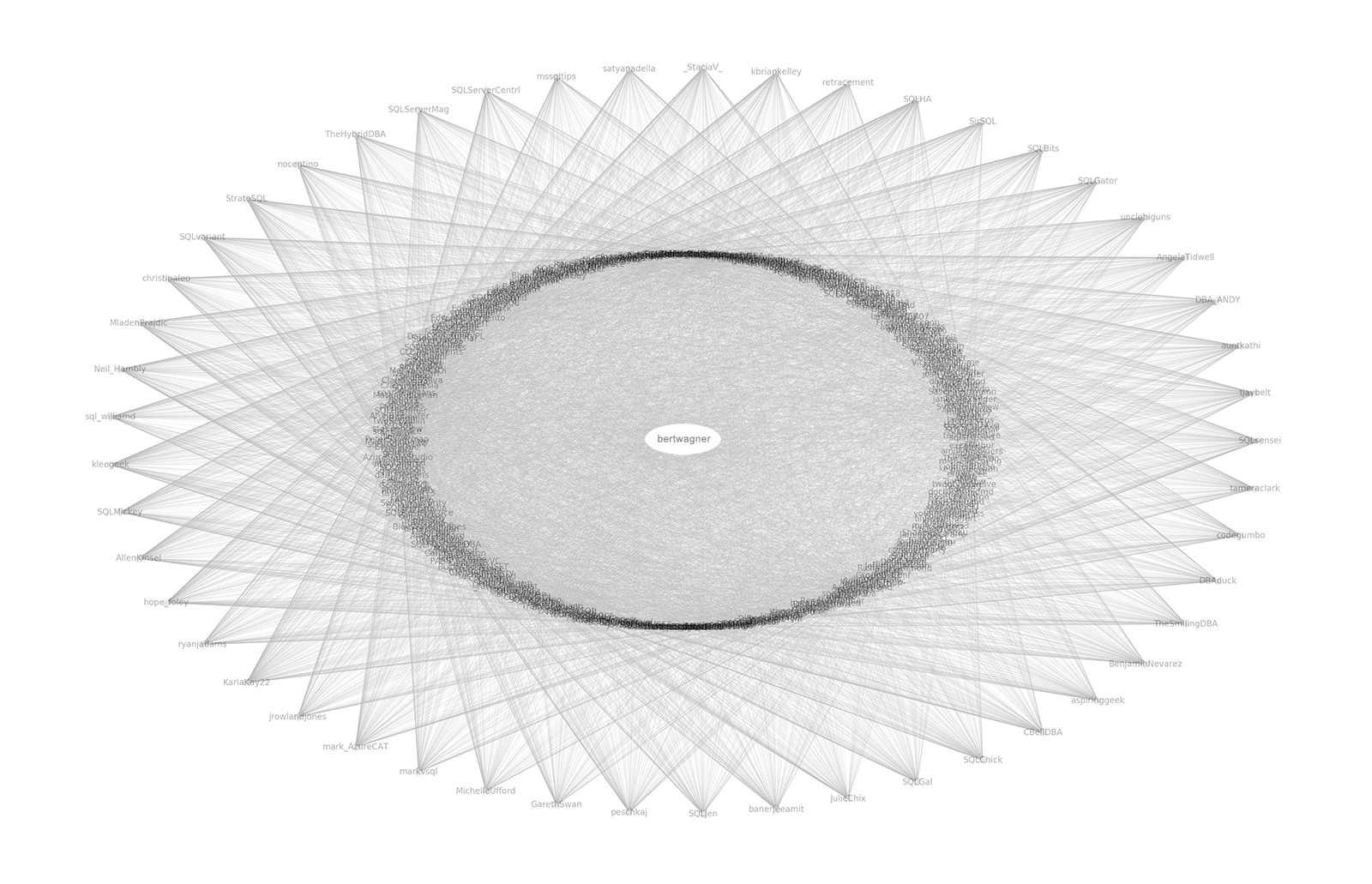
So that's it. It was fun using a graph library to find new people to follow on Twitter using the people I currently follow as a proxy for finding relevant users instead of Twitter's black box algorithms.